Llama Index.
Phiên bản của Phone và Typescript
Tài liệu của phiên bản Phone hoàn thiện hơn, ts kém hơn?
Vào đi.
Tạo môi trường
conda create --name llamaindex python=3.9.19
conda activate llamaindex
- Đặt môi trường Conda trong VS Code *
Python: Select Interpreter
Cài đặt thư viện
pip install llama-index pypdf sentence_transformers
Cấu hình OpenAI
vim ~/.bashrc
Thêm các biến môi trường
export OPENAI_API_KEY="sk-xxxx"
khứ cô.
echo $OPENAI_API_KEY
- Tình dục có thể * *
Cấu hình dòng lệnh: gproxy
Bắt đầu nhanh
import os.path
from llama_index import (
VectorStoreIndex,
SimpleDirectoryReader,
StorageContext,
load_index_from_storage,
)
import logging
import sys
logging.basicConfig(stream=sys.stdout, level=logging.DEBUG)
logging.getLogger().addHandler(logging.StreamHandler(stream=sys.stdout))
# check if storage already exists
PERSIST_DIR = "./storage"
if not os.path.exists(PERSIST_DIR):
# load the documents and create the index
documents = SimpleDirectoryReader("data").load_data()
index = VectorStoreIndex.from_documents(documents)
# store it for later
index.storage_context.persist(persist_dir=PERSIST_DIR)
else:
# load the existing index
storage_context = StorageContext.from_defaults(persist_dir=PERSIST_DIR)
index = load_index_from_storage(storage_context)
# either way we can now query the index
query_engine = index.as_query_engine()
response = query_engine.query("What did the author do growing up?")
print(response)
- Phương pháp compleons được sử dụng * *
/chat/completions
-
- Tham số để truy vấn * *
{
"messages": [
{
"role": "system",
"content": "You are an expert Q&A system that is trusted around the world.\nAlways answer the query using the provided context information, and not prior knowledge.\nSome rules to follow:\n1. Never directly reference the given context in your answer.\n2. Avoid statements like \"Based on the context, ...\" or \"The context information ...\" or anything along those lines."
},
{
"role": "user",
"content": "xxx"
}
],
"model": "gpt-3.5-turbo",
"stream": false,
"temperature": 0.1
}
- System Prompt* *
You are an expert Q&A system that is trusted around the world.
Always answer the query using the provided context information, and not prior knowledge.
Some rules to follow:
1. Never directly reference the given context in your answer.
2. Avoid statements like "Based on the context, ..." or "The context information ..." or anything along those lines.
Bạn là một hệ thống giải đáp chuyên gia được cả thế giới tin tưởng. Luôn sử dụng thông tin nền cung cấp khi trả lời các câu hỏi, thay vì kiến thức trước đây. Một số quy tắc cần tuân thủ:
1... Hay "thông tin nền cho thấy,..." Hay bất cứ cái đồng hồ tương tự nào.
-
- User Prompt* *
Context information is below.
---------------------
file_path: data/paul_graham_essay.txt
xxx
---------------------
Given the context information and not prior knowledge, answer the query.
Query: What did the author do growing up?
Answer:
Ứng dụng cảnh
应用场 | 说明 |
---|---|
Q&A | 最重要 |
Chatbots | |
Agents | 高级 |
Structured Data Extraction | 有用,整理聊天记录等 |
Multi-modal |
Nguyên lý cơ bản
Quy trình cơ bản
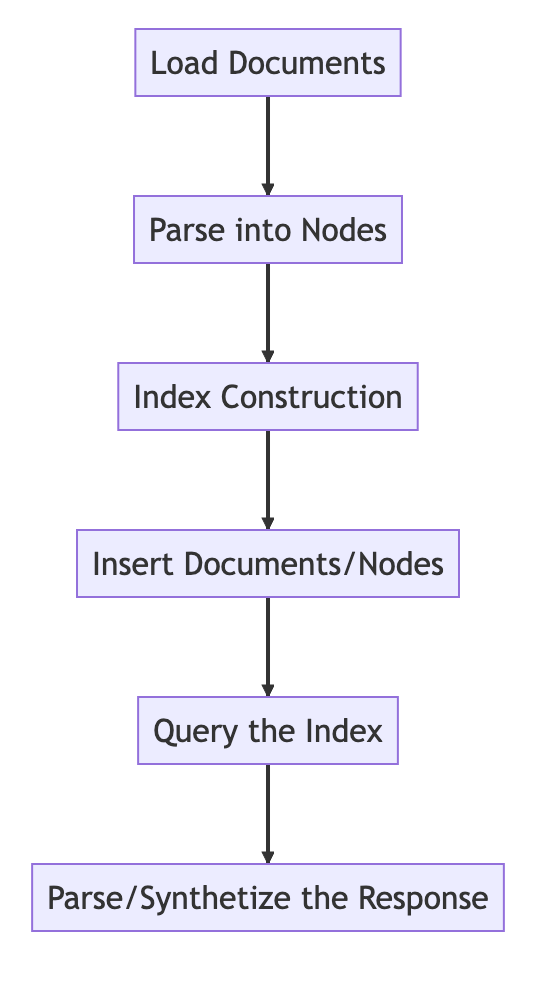
from llama_index import VectorStoreIndex, SimpleDirectoryReader
# Load in data as Document objects
documents = SimpleDirectoryReader('data').load_data()
# 切片,转成Node
# Parse Document objects into Node objects to represent chunks of data
index = VectorStoreIndex.from_documents(documents)
# Index Construction:创建索引
# Build an index over the Documents or Nodes
query_engine = index.as_query_engine()
# The response is a Response object containing the text response and source Nodes
summary = query_engine.query("What is the text about")
print("What is the data about:")
print(summary)
Chonking và Node
Dữ liệu nguồn -> Documents -> Nodes
(Accuments): chứa thông tin chính tả và tta
ID Document
Acument thực chất là loại phụ của Node
Lạ thật, một tập hồ sơ sẽ được cắt thành nhiều document.
Text Node: Dùng Node Parser để cắt Docutment thành nhiều hệ số Node
Nhật ký chứa Document
Node có mối quan hệ kết nối trước đó với Node
1.Node Parser nhận danh sách các đối tượng Document; 2... Phân chia văn bản của từng tài liệu với câu của Spy; 3... 4.Text Node bao gồm văn bản câu, và siêu dữ liệu như ID tài liệu, vị trí trong tài liệu; 5. Quay lại danh sách các đối tượng Text Node.
Lưu Document và index
hai cách thức / hai cách thức
- Lưu đĩa địa phương.
- Lưu trữ cơ sở dữ liệu.
- Đã lưu lại đĩa *
import os.path
from llama_index import (
VectorStoreIndex,
SimpleDirectoryReader,
StorageContext,
load_index_from_storage,
)
import sys
# check if storage already exists
PERSIST_DIR = "./storage"
if not os.path.exists(PERSIST_DIR):
# 保存数据: Load the documents and create the index
documents = SimpleDirectoryReader("data").load_data()
index = VectorStoreIndex.from_documents(documents)
# store it for later
index.storage_context.persist(persist_dir=PERSIST_DIR)
else:
# 从磁盘加载回数据: load the existing index
storage_context = StorageContext.from_defaults(persist_dir=PERSIST_DIR)
index = load_index_from_storage(storage_context)
query_engine = index.as_query_engine()
response = query_engine.query("What did the author do growing up?")
print(response)
Tạo chỉ mục
Tạo Embedding cho mỗi Node
Tạo chỉ mục ở Vector Strol Index
1... Một chỉ mục cũng lưu trữ các siêu dữ liệu trên mỗi nút như ID, vị trí, v.v... 3... Nút có thể truy xuất nội dung của một tài liệu hoặc tài liệu cụ
Chỉ mục truy vấn
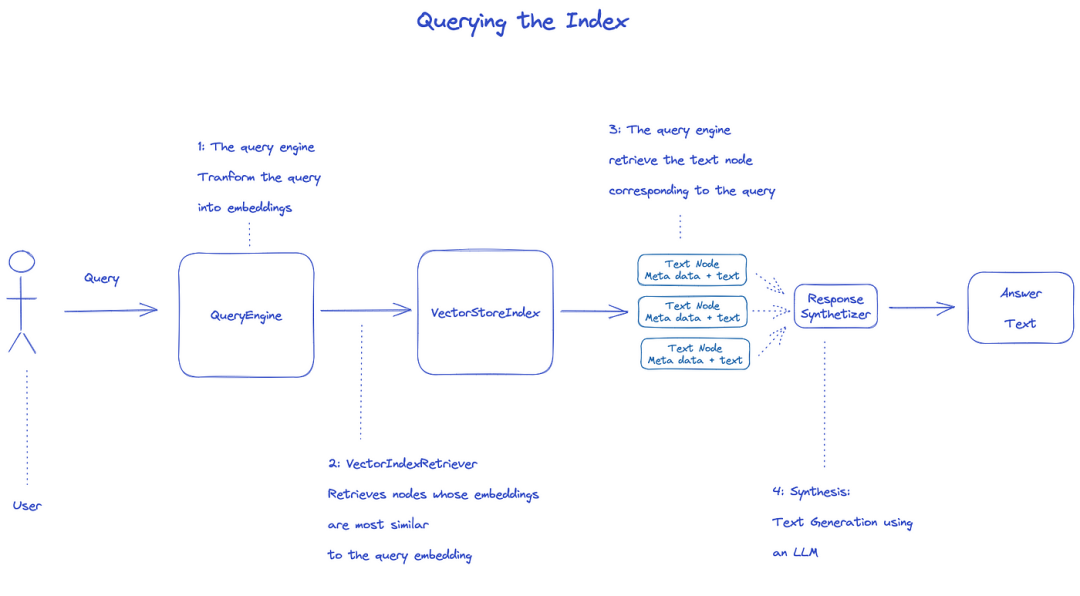
Để truy vấn chỉ mục, sẽ sử dụngQueryEngine.
- Retriever lấy các nút liên quan từ chỉ mục truy vấn. Ví dụ, Vector Index Retriever truy xuất các nút giống nhất với truy vấn Embedding; 2... Danh sách các nút được lấy lại được gửi tới Response Synsizer để tạo ra đầu ra cuối cùng; 3... 4.LLM nhập truy vấn và văn bản nút để có đầu ra cuối cùng;
from llama_index import (
VectorStoreIndex,
get_response_synthesizer,
)
from llama_index.retrievers import VectorIndexRetriever
from llama_index.query_engine import RetrieverQueryEngine
from llama_index.postprocessor import SimilarityPostprocessor
from llama_index import StorageContext, load_index_from_storage
# rebuild storage context
storage_context = StorageContext.from_defaults(persist_dir="storage")
# load index
index = load_index_from_storage(storage_context)
# configure retriever
retriever = VectorIndexRetriever(
index=index,
similarity_top_k=10,
)
# configure response synthesizer
response_synthesizer = get_response_synthesizer()
# assemble query engine
query_engine = RetrieverQueryEngine(
retriever=retriever,
response_synthesizer=response_synthesizer,
node_postprocessors=[SimilarityPostprocessor(similarity_cutoff=0.7)],
)
# query
response = query_engine.query("What did the author do growing up?")
print(response)
Tài liệu chính thức: Understanding
- 3 quy trình xử lý dữ liệu * *
Data / feature in in ML, eTL trong dữ liệu data / feature / enginion = data « dữ liệu » trong ML « ». . .
Ý tôi là, chuyện này có thể xảy ra với một người đàn ông như thế này. . .
- Load data
- Thay đổi dữ liệu
- Ấn tượng và dữ liệu.
Nạp dữ liệu (Ingestion)
-
Mục tiêu: * Định dạng các loại dữ liệu thành các đối tượng .
-
Nhập vào: * Dữ liệu đủ loại
-
Xuất: * Đối tượng
** 3 cách *
- Sử dụng các loại dương xỉ: thuận tiện nhất.
- Llama Huế: Những dụng cụ đã được viết sẵn.
- Tạo chữ ký hiệu.
** SimpleDirectoryReader
lớp **
from llama_index import SimpleDirectoryReader
documents = SimpleDirectoryReader("./data").load_data()
ủng hỗ trợ Markdon, PDFs, Worddocments (.docx), Powerpoint decks, images (.jpg, .png), odio và video
♪
- Notion (
NotionPageReader
) - Google Docs (
GoogleDocsReader
) - Slack (
SlackReader
) - Discord (
DiscordReader
) - Apify Actors (
ApifyActor
). Can crawl the web, scrape webpages, extract text content, download files including.pdf
,.jpg
,.png
,.docx
, etc.这个可以爬虫
** Tạo tài liệu trực tiếp **
from llama_index.schema import Document
doc = Document(text="text")
Chuyển đổi dữ liệu
-
Lý do: * Tiện nghi và LLM sử dụng hiệu quả
-
Hành động cụ thể: *
- Phân chia phần mềm Doucument (chunking)
- Trích xuất siêu dữ liệu (extractting metata data)
- Embedding.
** Nhập vào: ** Node
- Xuất: *
API sau khi đóng cửa
Sử dụng phương pháp fom_docments () Vector Store Index documents ()
from llama_index import VectorStoreIndex
vector_index = VectorStoreIndex.from_documents(documents)
vector_index.as_query_engine()
- Cách tùy chỉnh các tham số *
Ý tưởng: Dùng inter Service Context để tùy chỉnh
text_splitter = SentenceSplitter(chunk_size=512, chunk_overlap=10)
service_context = ServiceContext.from_defaults(text_splitter=text_splitter)
index = VectorStoreIndex.from_documents(
documents, service_context=service_context
)
Các nguyên tử API
Chế độ sử dụng chuẩn
from llama_index import Document
from llama_index.embeddings import OpenAIEmbedding
from llama_index.text_splitter import SentenceSplitter
from llama_index.extractors import TitleExtractor
from llama_index.ingestion import IngestionPipeline, IngestionCache
# 加载数据源
documents = SimpleDirectoryReader("./data").load_data()
# 创建转换数据的工作流
# create the pipeline with transformations
pipeline = IngestionPipeline(
transformations=[
SentenceSplitter(chunk_size=25, chunk_overlap=0), # 分片
TitleExtractor(), # 提取Meta信息
OpenAIEmbedding(), # Embedding
]
)
# 执行流程,生成节点
# run the pipeline
nodes = pipeline.run(documents=documents)
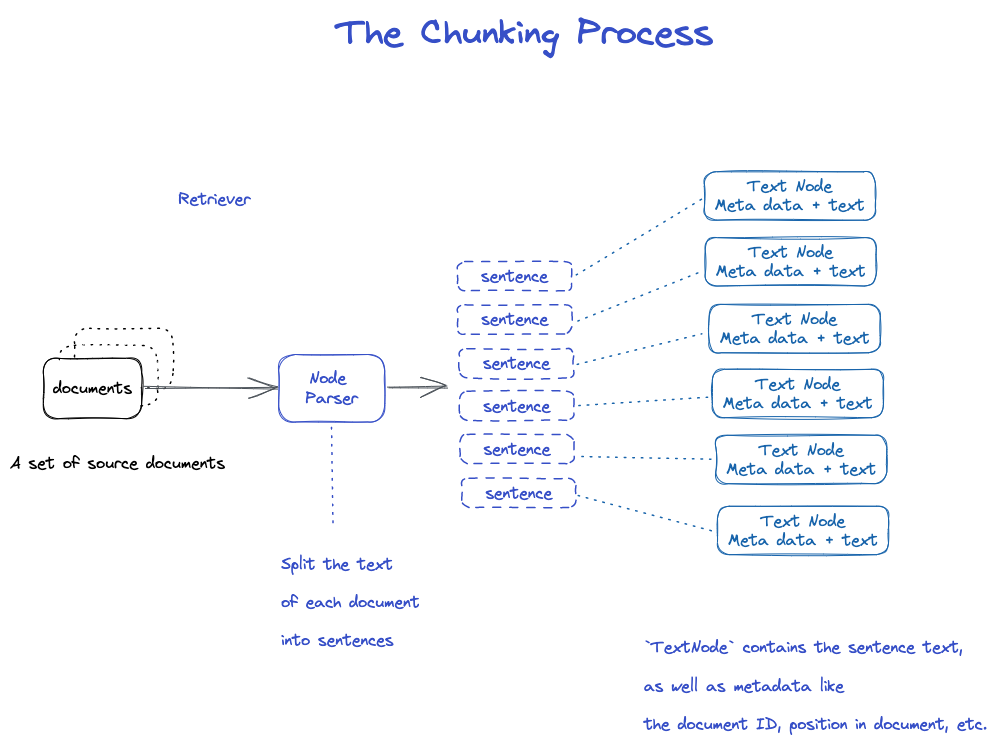
Chia ra.
Có rất nhiều chiến lược để xem cụ thể hơn một module Node Parser.
Thêm siêu dữ liệu
Bạn có thể tùy chỉnh tài liệu và nút, thêm siêu dữ liệu.
Tạo trực tiếp đối tượng Node
from llama_index.schema import TextNode
node1 = TextNode(text="<text_chunk>", id_="<node_id>")
node2 = TextNode(text="<text_chunk>", id_="<node_id>")
index = VectorStoreIndex([node1, node2])
Chỉ mục (index)
-
- Phân loại chỉ mục *
- Vector Stores
- Document Stores
- Index Stores
- Key-Value Stores
- Using Graph Stores
- [ Chat Stores] (
- Chỉ mục thường thấy * *
- Summary Index.
- Vector Store Index (phổ biến nhất)
- Được rồi.
- Keyword Table Index.
♪ Summary Index ♪ ♪

- Vector Store * *

** Chỉ số cây **
-
- Keyword Table Index *

- Cái gì?
Thêm Meta
document.metadata['lang'] = lang
Tử hình.
from llama_index.core.vector_stores import (
ExactMatchFilter,
MetadataFilters,
MetadataFilter,
)
filters = MetadataFilters(
filters=[
MetadataFilter(key="post_year", value="2017"),
],
)
# You pass filter as an argument. You can have any type of filter
# we saw above and then pass it to query engine.
query_engine = index.as_query_engine(service_context=service_context,
similarity_top_k=5,
filters = filters,
response_mode='tree_summarize')
response = query_engine.query("Marathon Running")
print(response)
Rsponse
- refine: Các câu trả lời liên tục với các context; trước hết hãy sử dụng mẫu text_qa_template, sau đó dùng mẫu refine_template. Mặc định. Tương tự refine, tuy nhiên, sẽ nhồi nhét context đầy yêu cầu một lần.
- Được rồi.
- Summaze.
Mã nguồn
Tài liệu
Document
Bao gồm:
-
Cái gì?
-
Làm ơn đi.
-
relations
: mối quan hệ với các tài liệu / nút khác
- Quy trình sử dụng nguyên tử *
from llama_index import Document, VectorStoreIndex
# 数据源
text_list = [text1, text2, ...]
# 手动创建documents
documents = [Document(text=t) for t in text_list]
# 建立索引: 传入document,在VectorStoreIndex再转换:分片转成Node,Embedding等
index = VectorStoreIndex.from_documents(documents)
Một số cách để tạo một Doument
- Tạo bằng tay *
from llama_index import Document
text_list = [text1, text2, ...]
documents = [Document(text=t) for t in text_list]
- Dùng data load (connector) *
Tất cả đều có một phương pháp là load_data ()
from llama_index import SimpleDirectoryReader
documents = SimpleDirectoryReader("./data").load_data()
** Tự động tạo dữ liệu mẫu **
document = Document.example()
Tự chọn Meta
from llama_index import Document
from llama_index.schema import MetadataMode
document = Document(
text="This is a super-customized document",
metadata={
"file_name": "super_secret_document.txt",
"category": "finance",
"author": "LlamaIndex",
},
excluded_llm_metadata_keys=["file_name"],
metadata_seperator="::",
metadata_template="{key}=>{value}",
text_template="Metadata: {metadata_str}\n-----\nContent: {content}",
)
print(
"The LLM sees this: \n",
document.get_content(metadata_mode=MetadataMode.LLM),
)
print()
print(
"The Embedding model sees this: \n",
document.get_content(metadata_mode=MetadataMode.EMBED),
)
Xuất
The LLM sees this:
Metadata: category=>finance::author=>LlamaIndex
-----
Content: This is a super-customized document
The Embedding model sees this:
Metadata: file_name=>super_secret_document.txt::category=>finance::author=>LlamaIndex
-----
Content: This is a super-customized document
Metada Extraction Usage Pattern (không hiểu)
Node.
Bản chất:các phần chia của Document
Làm thế nào để nhận được:
- Sử dụng lớp NodeParser để chuyển đổi tài liệu thành Node
- Tạo bằng tay
Cũng giống như Document, có:
-
Cái gì?
-
Làm ơn đi.
-
relations
: mối quan hệ với các tài liệu / nút khác
Khi chuyển từ Document sang Node, sẽ kế thừa các thông tin như Metadata.
Node là công dân số 1 trong Llama Index.
- Quy trình sử dụng nguyên tử *
from llama_index.node_parser import SentenceSplitter
# load documents
...
# 手动转换:切片,转成Node
# parse nodes
parser = SentenceSplitter()
nodes = parser.get_nodes_from_documents(documents)
# build index
index = VectorStoreIndex(nodes)
- Đặt quan hệ *
from llama_index.schema import TextNode, NodeRelationship, RelatedNodeInfo
node1 = TextNode(text="text_chunk1", id_="node_id1")
node2 = TextNode(text="text_chunk2", id_="node_id2")
node3 = TextNode(text="text_chunk3", id_="node_id3")
# set relationships
node1.relationships[NodeRelationship.NEXT] = RelatedNodeInfo(
node_id=node2.node_id
)
node2.relationships[NodeRelationship.PREVIOUS] = RelatedNodeInfo(
node_id=node1.node_id
)
node2.relationships[NodeRelationship.PARENT] = RelatedNodeInfo(
node_id=node3.node_id, metadata={"key": "val"}
)
print(node2)
Node Parser
Các mục đích: chuyển nguồn dữ liệu thành các đối tượng Node
Cụ thể: Phân chia một nhóm đối tượng Document thành nhiều đối tượng Node
Sự thực hiện cụ thể của thông thường
Node Parser là một loại trừu tượng, cụ thể thực hiện có:
** Theo loại tài liệu **
- SimpleFile Node Parser.
- HTML Node Parser.
- JSONod Parser.
- Markdon Node Parser.
-
- Chia văn bản * *
- Cái gì?
- Lanchrin Node Parser.
- Câu Splitter
- Sentence Window Node Parser (không hiểu)
- Semantic Splitter Node Parser (không hiểu, cảm thấy cao cấp hơn)
- Token Text Splitter.
** Mối quan hệ cha con**
- HierarchicalNodeParser: Sử dụng trong AutoMergingRetriever
cách sử dụng điển hình
- Dùng nguyên tử *
from llama_index import Document
from llama_index.node_parser import SentenceSplitter
# 创建NodeParser
node_parser = SentenceSplitter(chunk_size=1024, chunk_overlap=20)
# 调用 get_nodes_from_documents() 方法
# show_progress 可以显示进度
nodes = node_parser.get_nodes_from_documents(
[Document.example(), Document.example()], show_progress=True
)
print(len(nodes))
print()
print(nodes[0])
Xuất
2
Node ID: eaeb6e44-6828-4e36-b7a3-69342de4dc7c
Text: Context LLMs are a phenomenal piece of technology for knowledge
generation and reasoning. They are pre-trained on large amounts of
publicly available data. How do we best augment LLMs with our own
private data? We need a comprehensive toolkit to help perform this
data augmentation for LLMs. Proposed Solution That's where LlamaIndex
comes in. Ll...
- Transformations * ♪
from llama_index import Document
from llama_index.node_parser import SentenceSplitter
from llama_index.ingestion import IngestionPipeline
from llama_index.node_parser import TokenTextSplitter
documents = [Document.example(), Document.example()]
# 创建NodeParser
node_parser = SentenceSplitter(chunk_size=1024, chunk_overlap=20)
# 将NodeParser放到Pipeline中的transformations列表
pipeline = IngestionPipeline(transformations=[node_parser])
nodes = pipeline.run(documents=documents)
print(len(nodes))
print()
print(nodes[0])
- Dùng Service Context *
from llama_index import Document, ServiceContext, VectorStoreIndex
from llama_index.node_parser import SentenceSplitter
from llama_index.ingestion import IngestionPipeline
from llama_index.node_parser import TokenTextSplitter
documents = [Document.example(), Document.example()]
node_parser = SentenceSplitter(chunk_size=1024, chunk_overlap=20)
service_context = ServiceContext.from_defaults(text_splitter=node_parser)
index = VectorStoreIndex.from_documents(
documents, service_context=service_context, show_progress=True
)
Ý tôi là...
Đầu vào: một nhóm các node
Xuất:các nhóm Node
Có hai phương pháp công cộng:
- _ call__ () đồng bộ: đồng bộ
- ng nh
Node Parser và Metadata Extractor thuộc sở hữu của Transformations
** Sử dụng chế độ **
from llama_index.text_splitter import SentenceSplitter
from llama_index.extractors import TitleExtractor
node_parser = SentenceSplitter(chunk_size=512)
extractor = TitleExtractor()
# use transforms directly
nodes = node_parser(documents)
# or use a transformation in async
nodes = await extractor.acall(nodes)
- Dùng kết hợp với Service Context * *
from llama_index import ServiceContext, VectorStoreIndex
from llama_index.extractors import (
TitleExtractor,
QuestionsAnsweredExtractor,
)
from llama_index.ingestion import IngestionPipeline
from llama_index.text_splitter import TokenTextSplitter
transformations = [
TokenTextSplitter(chunk_size=512, chunk_overlap=128),
TitleExtractor(nodes=5),
QuestionsAnsweredExtractor(questions=3),
]
# 创建ServiceContext,传入Transfrmation
service_context = ServiceContext.from_defaults(
transformations=[text_splitter, title_extractor, qa_extractor]
)
# 传入VectorStoreIndex的from_documents()或insert()方法
index = VectorStoreIndex.from_documents(
documents, service_context=service_context
)
Service Context
một gói các dịch vụ và cấu hình được sử dụng trên một đường ống LlamaIndex.
- Có thể cấu hình *
from llama_index import (
ServiceContext,
OpenAIEmbedding,
PromptHelper,
)
from llama_index.llms import OpenAI
from llama_index.text_splitter import SentenceSplitter
# 设置LLM
llm = OpenAI(model="text-davinci-003", temperature=0, max_tokens=256)
# 设置Embedding模型
embed_model = OpenAIEmbedding()
# 设置Chunk的大小
text_splitter = SentenceSplitter(chunk_size=1024, chunk_overlap=20)
prompt_helper = PromptHelper(
context_window=4096,
num_output=256,
chunk_overlap_ratio=0.1,
chunk_size_limit=None,
)
service_context = ServiceContext.from_defaults(
llm=llm, # 设置LLM
embed_model=embed_model, # 设置Embedding模型
text_splitter=text_splitter, # 设置Chunk的大小
prompt_helper=prompt_helper,
)
- Kiến tạo hàm truyền nhân * (dễ dàng hơn)
♪
- Xin lỗi.
- Xin lỗi.
♪ ♪
- ng:
- Không, không.
Ví dụ như
service_context = ServiceContext.from_defaults(chunk_size=1000)
-
- Cấu hình toàn cục * *
from llama_index import set_global_service_context
set_global_service_context(service_context)
-
- Cấu hình địa phương * *
query_engine = index.as_query_engine(service_context=service_context)
Storage Context
Hậu quả của sự việc xảy ra ở đâu, và indexs đang ở đâu? >> index indexs / index indexs ♪
[API Tài liệu tham khảo] (https://docs.llamaindex.ai/en/stable/api_reference/)
store = PGVectorStore(
connection_string=conn_string,
async_connection_string=async_conn_string,
schema_name=PGVECTOR_SCHEMA,
table_name=PGVECTOR_TABLE,
)
index = VectorStoreIndex.from_vector_store(store)
Vector, Store ấn tượng.
Các hàm cấu trúc
index = VectorStoreIndex.from_vector_store(store)
Engine có hai loại:
- Query Engine: BaseQueryEngine
- Chat Engines: BaseChatEngine
Tạo Engine
index.as_query_engine()# BaseQueryEngine
index.as_query_engine(streaming=True)# 流式 BaseQueryEngine
index.as_chat_engine() # BaseChatEngine; 流式不是在这里控制
Các truy vấn
# Query
response = await query_engine.aquery(query) # 流式
response = await query_engine.aquery(query)
# Chat
response = await chat_engine.astream_chat(last_message_content, messages) # 流式在这里控制
response = await chat_engine.achat(last_message_content, messages)
BaseQueryEngine
- aquery
Base ChatEngine
-chat
- stream_chat
- Mua
- stream_chat
Tiếp tục hỗ trợ dòng chảy: stylist
hỗ trợ các bước lạ: a đầu
Loại phản hồi
# Query
RESPONSE_TYPE = Union[
Response,
StreamingResponse, AsyncStreamingResponse, #流式
PydanticResponse
]
# Chat
StreamingAgentChatResponse #流式
AGENT_CHAT_RESPONSE_TYPE = Union[AgentChatResponse, StreamingAgentChatResponse] #非流式
Làm thế nào để đối phó với dòng chảy
Giao diện tiêu chuẩn sử dụng Python:
- StreamingResponse() -AsyncStreamingResponse
- Dreaming Response
- Chào.
@r.post("")
async def chat(
request: Request,
queryData: _QueryData,
query_engine: BaseQueryEngine = Depends(get_query_engine_stream),
):
query = queryData.query
streaming_response = await query_engine.aquery(query)
async def event_generator():
async for token in streaming_response.async_response_gen:
if await request.is_disconnected():
break
yield f"data: {token}\n\n"
return StreamingResponse(event_generator(), media_type="text/event-stream")
- Chat.
@r.post("")
async def chat(
request: Request,
data: _ChatData,
chat_engine: BaseChatEngine = Depends(get_chat_engine),
):
last_message_content, messages = await parse_chat_data(data)
response = await chat_engine.astream_chat(last_message_content, messages)
async def event_generator():
async for token in response.async_response_gen():
if await request.is_disconnected():
break
yield token
return StreamingResponse(event_generator(), media_type="text/plain")
StreamingTrả lời
class AsyncStreamingResponse:
async_response_gen: TokenAsyncGen
class StreamingResponse:
response_gen: TokenGen
Rsponse
Giám sát.
Giáo trình.
Hướng dẫn về Deeplearn
Building and Evaluating Advanced RAG Applications:链接 Bilibili
**Join Text to SQL và Semantic Search **
Video này bao gồm các công cụ được xây dựng trong LlamaIndex để kết hợp SQL và tìm kiếm ngữ nghĩa vào một giao diện truy vấn thống nhất duy nhất.